research Research programme Module 3
Module 3
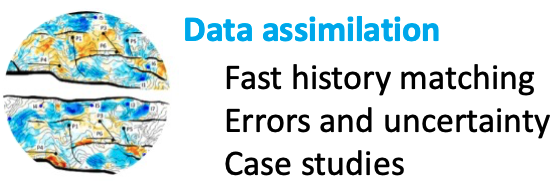
Data assimilation is defined as a technique whereby data are combined with output from numerical modelling to produce an optimal estimate of the evolving system. Generically, the description defines the process of history matching combined with forward prediction or forecast, and the central idea behind (seismic) history matching using 4D seismic data. In our case of course, the key idea is to ‘train’ model parameters based on the observed data. The model is a mathematical (geo-cellular) representation of the geology, and prediction is of fluid flow and pressure evolution during reservoir production and recovery. Currently, the ensemble method is a popular way of achieving this objective. With the evolution of ETLP’s MOPHiM v 2.0 (Meta-heuristic optimisation platform for history matching) our tool for implementing seismic history matching over Phase VII, our ability to implement a coherent SHM workflow over Phase VII and into Phase VIII has improved. This links data to simulations via a specific objective functions that can be optimised using several algorithms such as SHADE, differential evolution, CMA-ES and particle swarm optimisation. The code is written in Python and is written in an easy-to-use plugin in, plugout environment. This has provided new students and staff with a flexible environment to quickly assess new ways to create a model update.
At ETLP we still maintain that matching begins with direct updating, an ETLP procedure in which certain obvious information or low hanging fruit (static or dynamic – such as fault structure, transmissibility, barriers, sand body distribution) are extracted directly from the 4D seismic interpretations to help honour all domains of discipline (this may also be termed ‘model maturation’). We recommend that the convergence between the 4D seismic data, production data, and a suitable model representing future forward predictions must take place slowly and according to certain calibration stages or decision gates evolving as described by Wong et al. (2020) and the tiered integrated approach in Figure 18. This understanding has not significantly changed by the research in Phase VII, and we continue to apply this approach to a range of datasets.
In Phase VIII we recognise three main topic themes which we believe are essential to make future progress in this subject: speeding up the time to reach an optimal point (fast history matching and model update), efficient capture of the errors and uncertainty, and finally the need to apply our methods to practical case studies.
Fast history matching
Direct updating of the simulation model and assisted seismic history matching are known to be time-consuming processes. This is an obvious bottleneck in the workflow processes and still need to be addressed by future research. Here we investigate new ways to provide an update by providing faster ways of assimilating the 4D seismic data into our models, to help close the small loop between the geophysicist and reservoir engineer. In Phase VII, to achieve speed with robustness we reached back into the past to discover some innovative ideas for developing proxies to the simulation model (MacBeth et al. 2016). Work by Chong (2018) considered several proxies for the simulation model suited to seismic history matching, whilst Zheng (2019) explored the possibilities of using machine learning to forecast production. Before 3D numerical simulation emerged, engineers were very inventive with the way they simulated the reservoir using mechanistic, empirical, data- driven or generalized physics-based models. We found approaches such as the map-based transform, flat areal models, or a tank-representation as beneficial. Other proxies to the full numerical simulation model include water or gas front prediction, polynomial regression model, or the use of well know streamline simulation.
One of the main differences between the start of Phase VII and Phase VIII is the recent rise in popularity of AI, and in particular deep learning. Thus, the design of suitable proxy boils down to network architecture choice and application (for example: Yu et al. 2008; Cullick et al. 2016; Santos et al. 2020). Apart from replacing the non-linear response surface from the simulator, machine learning can also be used for dimensionality reduction in the input seismic, the actual optimisation procedure, data-driven production forecast from the well (for example Boomer 1995) and 4D seismic data, and replication of the PEM or sim2seis procedure. Clearly there are pros and cons of each option, which we intend to explore during this phase. For the purposes of this study we are also exploring new ways of visualising multi-dimensional solution space to support the history matching process.
The following will be considered:
- Machine learning proxy models for
- Simulation model
- PEM
- Production forecast
- Dimensionality reduction and optimisation
- Application to data from frequent seismic acquisitions
- Fast turnaround/best procedure and workflows
- General use of proxies to close the loop
We continue to explore and consolidate quick and efficient ways of reservoir model updating through direct or history-match procedures using our ever increasing stable of algorithms. In the updating, we look specifically at the issues of speed of turnaround as we acknowledge that the conventional history matching process is time consuming and yields non-unique answers. We find ways to better pursue the match to the seismic and, in particular, suitable seismic attributes to provide adequate match.
Errors and uncertainty
One over-arching principle has been identified by our sponsors during our various meetings – the need to specify uncertainties. This is true for the 4D seismic analysis, when describing different domains, workflows, and also with the seismic history match regardless of whether this is a direct update, wiggle trace, impedance domain, pressures and saturations, time shifts, or when utilizing the binary or ternary approach. Flags to understand the quality of the data input into the seismic history matching are of utmost importance. The use of error models to compare and evaluate our results remains a challenge. One project in Phase VII (Hatab 2019) has addressed this challenge (Figure 16) and will propagate this line of investigation in Phase VIII through the QI workflow.
ETLP recognises that there are a large list of disparate errors (fluid flow model realism, seismic modelling, data processing, wavelet, PEM, scale-related, etc..) that contribute to the final match between the predicted and the observed 4D seismic data (MacBeth 2010). Most of these errors are ignored in the popular practice of comparison using a mathematical objective function, for which an extensive array of literature has emerged. The groups of errors are also quite different between well data and seismic. A satisfactory treatment and mitigation of these uncertainties is vital (MacBeth et al. 2020) before the objective function can be explored or visualised by large and powerful search engines that search an extremely complex problem (for example, Oliver et al. 2008). Despite this backdrop, some success has been achieved by focusing on discrete features on the seismic such as contact movement or binarizing the data (Obidegwu et al. 2016, Mitchell and Chassagne 2019), and to a limited extent with ternary representation (Zhang et al. 2018). SHM has been observed to work best in practice by manual and visual comparison, but currently lacks the ability for accurate quantitative comparison.
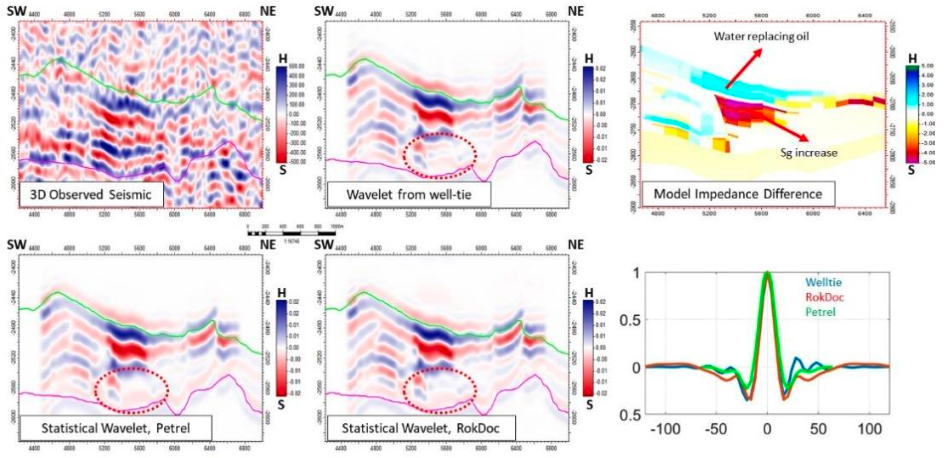
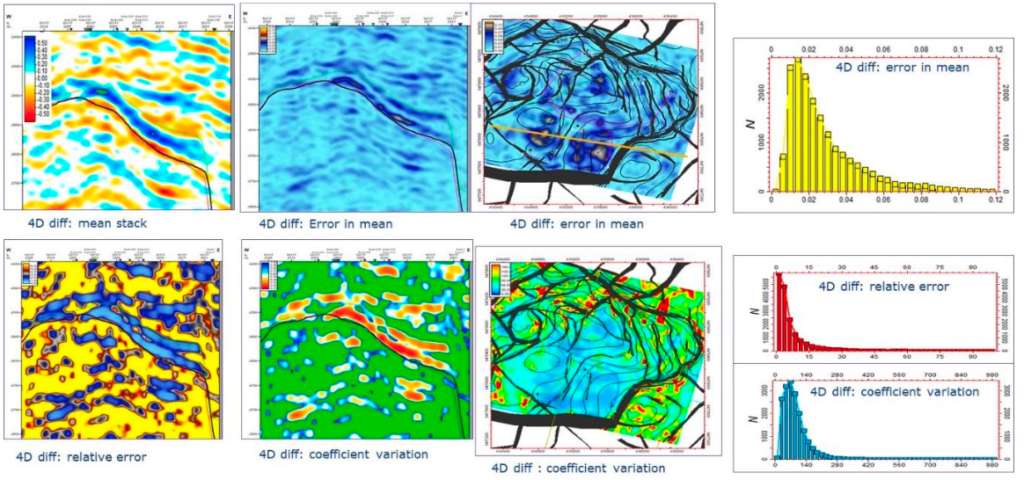
To date these errors (contributing to both a bias and uncertainty) have not been taken into account, although a study (Jan et al. 2019) has progressed in the investigation of many elements and this will continue to grow into Phase VIII. The work of Hatab et al. (2019) has shown that a sensitivity study of the pre-stack processing workflow is necessary to take proper account of errors in the observed data (see Figure 16). Future studies will aim to fully elaborate on the major cause of the mismatch between the simulator predictions and the 4D seismic data, and how these can be resolved based on a range of case-dependent applications. From these we hope to be able to make a statement on the likely errors on the parameters that are being history matched in the simulation model.
The following will be considered:
- Accumulation of errors from pre-stack workflow to processed data
- Quantification of errors in fluid flow modelling
- Schemes for assessing all errors in seismic history matching
- Data redundancy and necessity – how much is actually needed (binary versus ternary versus PCA)
- Application to field data, metrics for data quality
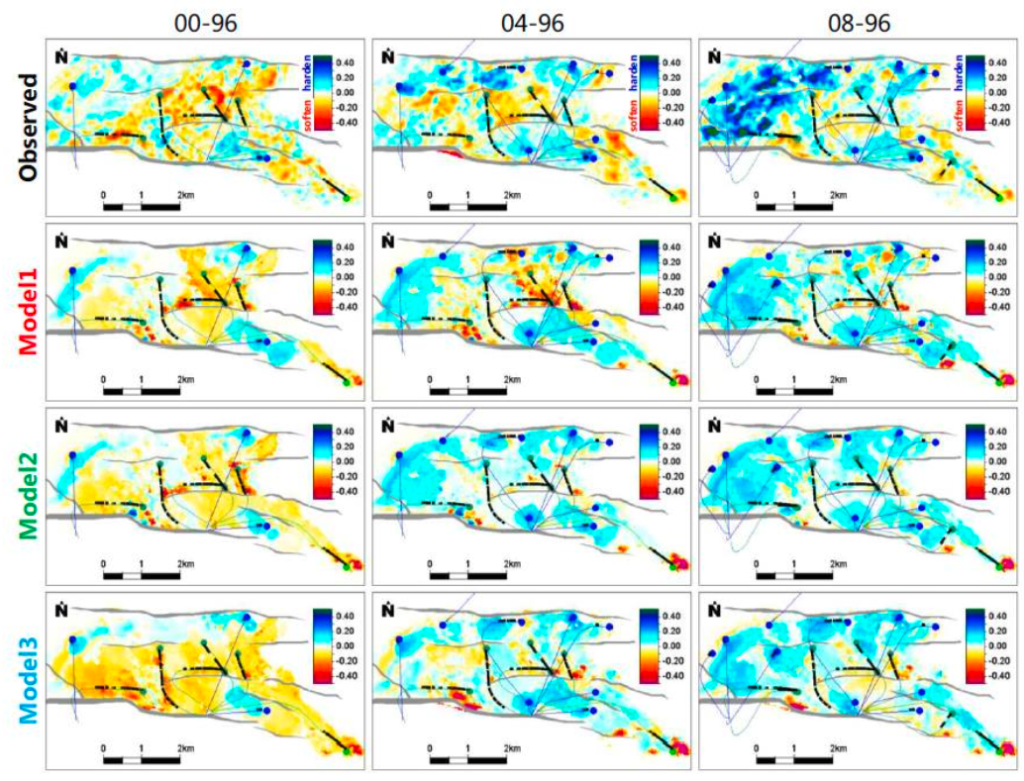
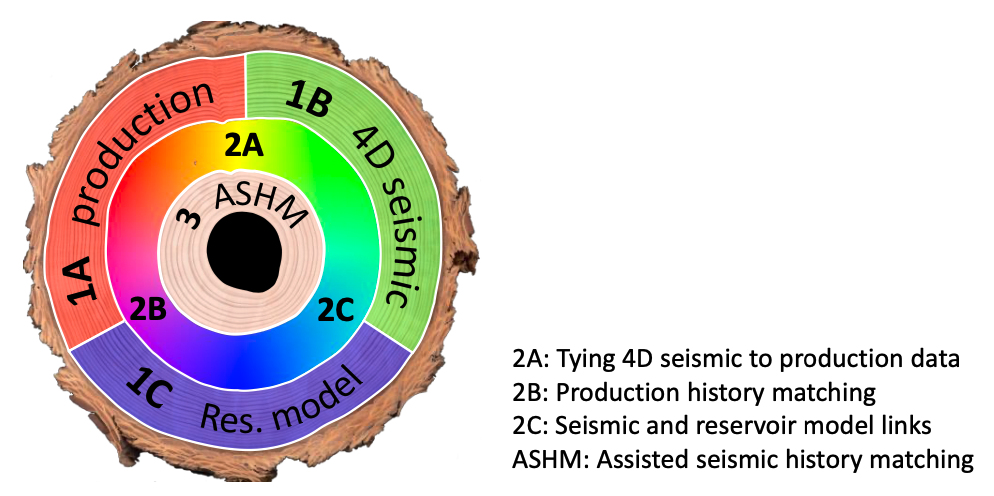
Case studies
Field datasets form the backbone of ETLP studies. The simulation model in particular is key to understanding the fluid flow and pressure variations during production and is essential for closing the loop with the 4D seismic data. We now have access to a wide range of simulation models which our reservoir engineering-polarised students will use as a starting point for their research studies. We recognise that a reliable well-history matched simulation model is also required to provide any chance of success in seismic history matching. Given our current datasets, this module will predominantly focus on the application of existing SHM and closing the loop techniques to different datasets and settings, to build up an understanding of where the need and requirement for the SHM arises. Thus, we will carry out a comparative study across a range of fields. Practical problems relating to field management issues will be catalogued for these fields, and solutions sought both via SHM and direct 4D seismic interpretation (see, for example, Hallam et al. 2019). For this the workflow shown in Figure 18 becomes important, as this relates each dataset to what can be calibrated using the field data. This is key as early examples of SHM over-stretched the applicability and were merely examples of the mathematical technology rather than useful workflows for practical reservoir management. SHM has not significantly improved in that respect, although tools for better comparison between the final results and the seismic data do exist (Hodgson et al. 2017, Amini et al. 2019) but is considered an elite tool or used in the final polishing step in the 4D analysis. Is it really needed, can it be avoided, or is it a necessary step in the workflow? These are some of the questions we intend to address in this sub-module. In all of the above, we seek to produce as many case studies as possible to fine-tune our generic approach and to mature our understanding of what is possible in this vital last stage of the 4D workflow journey.