research Research programme Module 2
Module 2
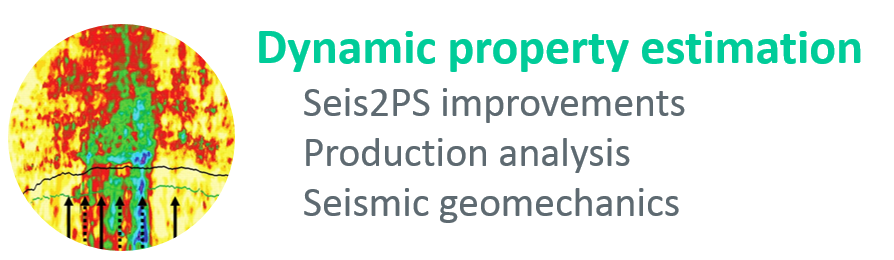
This module contains several well-recognised and popular topics from the 4D seismic and engineering world. We maintain our research effort on these topics because we believe that there are still unresolved issues and current understanding is not complete. For example, pressure-saturation separation (seis2P&S) is one of the keys to understanding the reservoir connectivity and dynamics and forms invaluable input into a seismic history match. Despite the maturity of estimation procedures much still remains to be achieved in terms of obtaining robust, high resolution estimates directly from 4D seismic signatures. We find that the production setting is important and hope by application of our insights to field datasets to be able to extend our methods and understanding to situations such as a stacked reservoir environment or structurally complex field settings. Within this module we also consider engineering-based studies such as well pressure interference tests, which we will attempt to link to the 4D seismic data either by deterministic algorithms or machine learning. Our hope is to recover an understanding of reservoir connectivity and transmissibility to act as an aid in field management. The third topic for consideration is the familiar seismic geomechanics, for which much remains to be done. Advances have been made in working with post-stack time shifts, however pre-stack TVO and AVO remain two additional dimensions to exploit to better understand the principal components of strain (and stresses) in the overburden and reservoir. The challenge here is to improve our current definition by careful inversion and modelling, and study of several geomechanically active datasets. We also focus on better understanding the R factor and defining a rock mechanical model for 4D seismic geomechanics interpretation.
Pressure and saturation estimation
To date, analyses have included AVO (restricted offset stacks), engineering consistent multi-attribute inversions, the use of inverted impedance changes and post-stack time- shifts. Recent advances also include stochastic inversion and machine learning applications (Corte et al. 2019). Here our intention is to continue this trajectory by considering more applications to clastic and chalk reservoirs. This will combine with a critical assessment of what we cannot and can detect using the 4D seismic monitoring technique. Our hope is to extend the applicability of the method to reservoir situations in which the 4D dynamic signals overlap. For example, the case of one producing reservoir unit overlying another, with variable thicknesses and reservoir quality. Examining ways of clearly separating the two responses on the amplitudes and time-shifts. Another interest is the estimation of the dynamic signals for recovery mechanisms that are complex and cyclical such as water alternating gas (WAG) (Figure ). The main issue is the vertical resolution of water and gas, detection and separation of the pressure and saturation signals. The aim is to improve areal and vertical sweep conformance by monitoring using the seismic data. The analysis will be complemented by sim2seis (simulator to seismic modelling) scenario analysis followed by seis2sim (inversion to simulator properties) and seis2imp inversion (inversion to impedances).
Themes include:
• Review and consolidation of state of science
• Pressure and saturation separation–stacked reservoirs
• Quantification of the WAG process
• Further development of machine learning for inversion
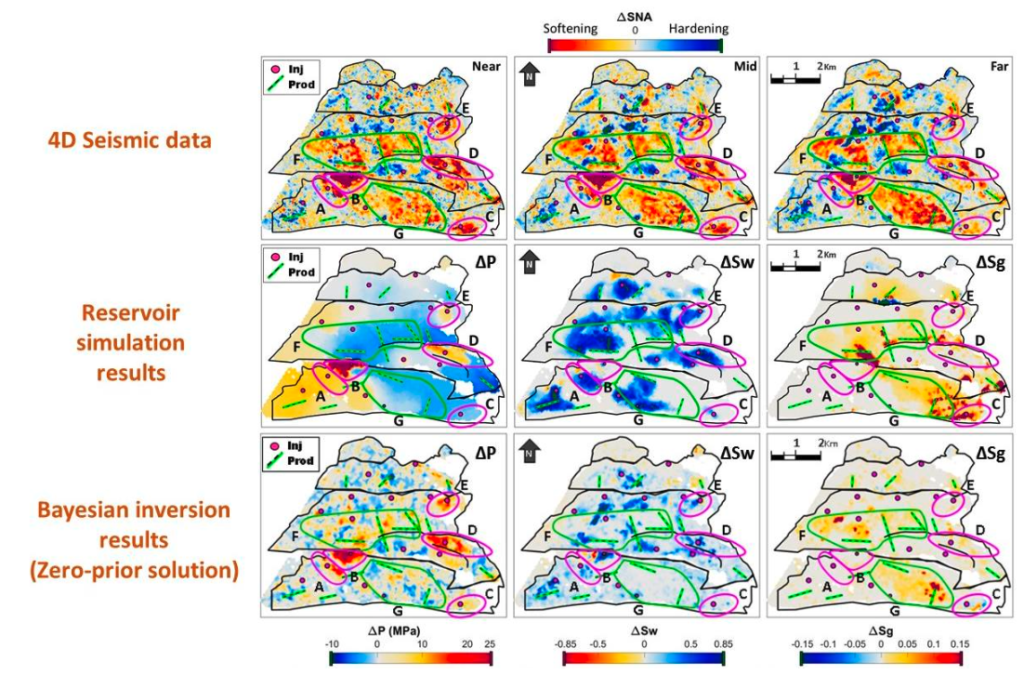
Connectivity analysis
Inferring inter-well connectivity using tracers, fluid rates and pressure data at the wells remains an important topic for production optimisation. For example, as pointed out by Tian and Horne (2016), in a waterflood the key issue is to locate injectors and adjust injection and production strategy on a well control level in order to optimise the producers. This requires a field-wide understanding of how the production is influenced by the injection. This knowledge may be acquired by multi-variant correlations, connectivity analyses such as that offered by the capacitance-resistance model of Albertoni and Lake (2003), tracer data, well to well interference tests, and many more well-based approaches which include the use of machine learning. Our previous work (Wong et al. 2019) has used the capacitance-resistance model to determine well to well correlations with the aim of integrating these results with 4D seismic data to enable pathways of communication to be defined for model update. These analyses also combine favourably with the highly successful well2seis approach developed by Huang et al. (2011) and further extended by Yin et al. (2015) to compare both well2well and well2seis. Our aim here is to continue to explore joint inversions of 4D seismic and non-seismic engineering data in this context. In this respect, machine learning may be used as a way of exploring the data correlations.
The following are the main points
- Engineering-based analysis of well data such as tracers, produced and injected volumes, pressure data, simulation, geological assessment of connectivity, production logging tool data
- Joint integrated interpretations between 4D seismic and the production data (Figure 12 shows one visual result after Yin et al. 2015)
- Use of the well2seis technique in combination with well2well approaches
- Application to a clastic and chalk field
- Application of machine learning techniques to implement the above
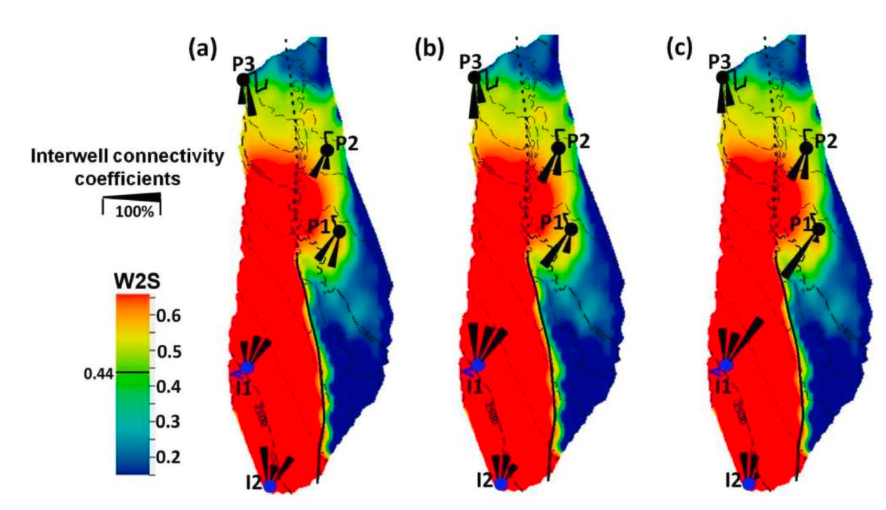
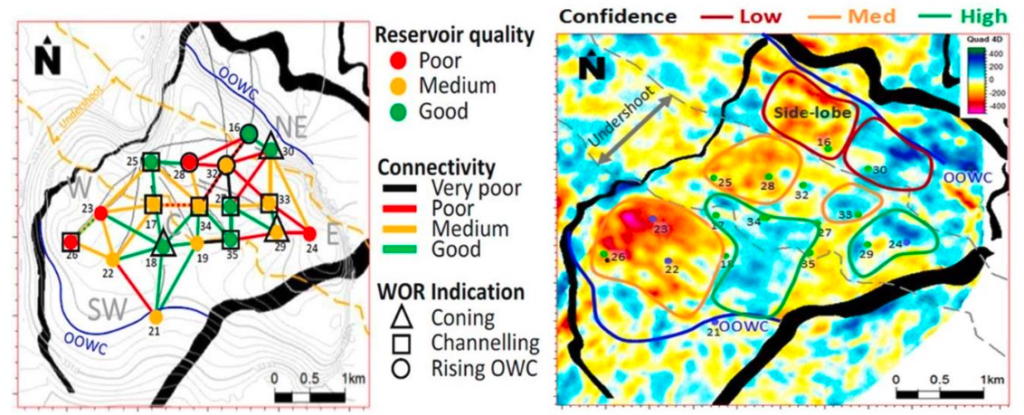
Seismic Geomechanics
The use of 4D seismic as a tool for interpreting geomechanical variations in the subsurface has become widespread in industry. ETLP research has evolved from 3D warping measuring vertical and lateral displacements (Hall and MacBeth 2001), time-shift estimation (see the review of MacBeth et al. 2020), to mech2seis modelling (Jaramillo et al. 2019). It is also possible to invert overburden time-shifts for reservoir pressure in clastic (Hodgson and MacBeth 2006; Garcia and MacBeth 2012) and chalk (Corzo et al. 2010; Wong et al. 2017) reservoirs. Close the loop exercises have been considered by Jaramillo (2019) for an HPHT field (see Figure 14). Whilst much work has already been accomplished in this area, and code already exists to implement these studies, there are still some outstanding issues. For example, lateral shifts (and hence the horizontal principal strains/stresses) have not been widely measured, and this may be perhaps due to the difficulty in obtaining an accurate measure. Also, many studies to date have worked with data shifts in the post—stack domain, and pre-stack amplitudes have not been investigated to determine their value. Further work to refine this analysis involves understanding the interplay between imaging shifts and those induced by the subsurface displacements. In this context, the role of imaging and wave-physics has been studied by several researchers in the past (Hoeber et al. 2016), but a practical tool for universal application remains to be found. This is particularly relevant as we consider data from fields with complex overburdens and structurally challenging reservoirs. One particular desire is to use additional non-seismic data in support of the 4D seismic geomechanical measurements such as subsidence, 4D gravity or microseismic.
Key features of this sub-module are therefore:
- Pre-stack amplitude variations
- Use of AVO to analyse the principal stresses/strains
- Measuring and modelling time-shifts or velocity changes, horizontal shifts, pre-stack data
- Time-shift variation with offset – interpretation and value
- Time-shift interpretation:
- Extension to reservoirs with complex overburdens
- Estimation of reservoir pressure using volumes of time-shifts
- 3D warping and residual imaging analysis (re-visited)
- imaging-based solutions, and close the loop modelling/inversion
- Case studies to close the loop between the geomechanical model and the 4D seismic
- Cross-learning into the CO2 project
- Enhanced mech2seis capability
- Machine learning to replace Geertsma or Green’s function approach to time-shift inversion
It is anticipated that there will be applications to datasets from HPHT and chalk reservoirs, although other data must also be considered.
